Optimizing for Resilient Critical Infrastructure
Contact: Prof. Giovanni Sansavini
In the last decade, resilience analysis has grown as a proactive approach to enhance the ability of infrastructures to prevent damage before disturbance events, mitigate losses during the events, and improve the recovery after the events. Yet, the concept of resilience is still evolving and there is no widely-accepted indicator to measure it in all its dimensions.
Assessing resilience of interdependent infrastructures
Our group developed a time-dependent metric for resilience quantification in infrastructures that integrates the multiple resilience capabilities, i.e., absorptive, adaptive, and restorative capabilities. Moreover, the metric allows measuring resilience in interdependent infrastructures made of diverse interconnected functional and physical layers, i.e., the so-called systems of systems. We exemplified this with reference to the Swiss electric power supply system. To this aim, we identified three main layers of interdependency, i.e. the bulk power system, the SCADA infrastructure and the human operator, and simulated them by means of agent-based, hybrid, multi-layer modeling. The results provide insights to decision makers for strengthening the resilience against failures and disruptions, i.e., by ranking the benefits of resilience improving strategies.
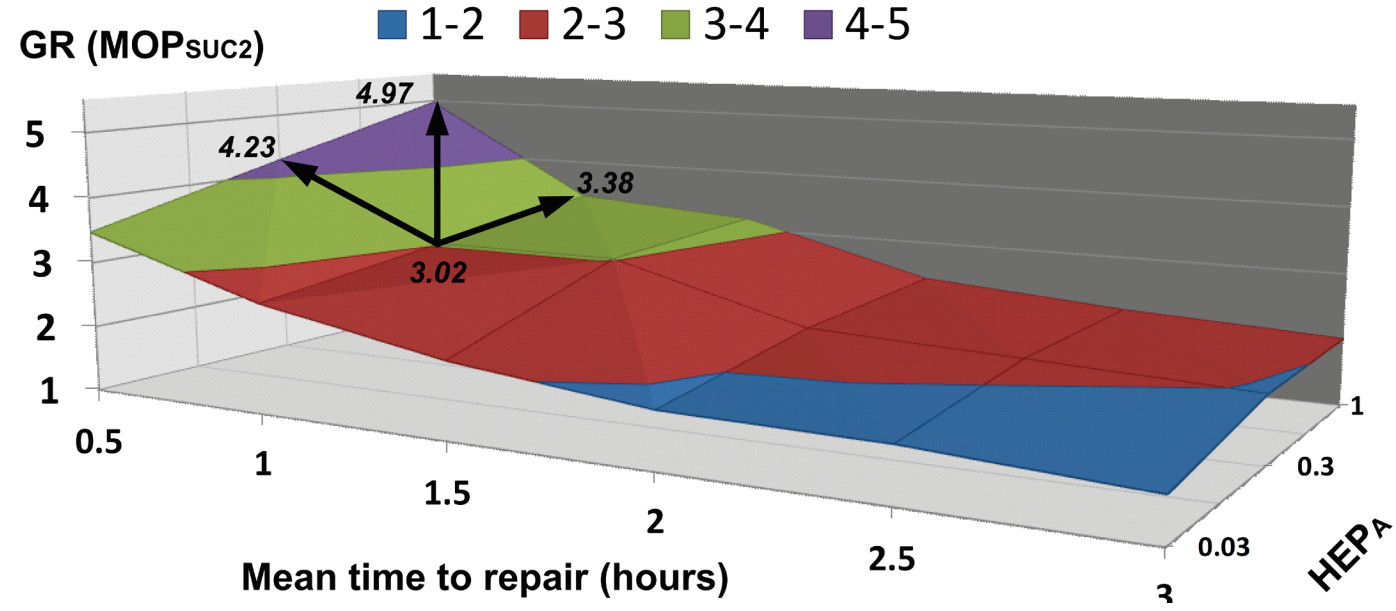
Optimizing power system investments and resilience against attacks
We study the combination of capacity expansion and switch installation in electric systems that ensures optimum performance under nominal operations and attacks. The planner–attacker–defender model is adopted to develop decisions that minimize investment and operating costs, and functionality loss after attacks. The model bridges long-term system planning for transmission expansion and short-term switching operations in reaction to attacks. The mixed-integer optimization is solved by decomposition via two-layer cutting plane algorithm. Numerical results on an IEEE system shows that small investments in transmission line switching enhance resilience by responding to disruptions via system reconfiguration. Sensitivity analyses show that transmission planning under the assumption of small-scale attacks provides the most robust strategy, i.e. the minimum-regret planning, if many constraints and limited investment budget affect the planning. On the other hand, the assumption of large-scale attacks provides the most robust strategy if the planning process involves large flexibility and budget.
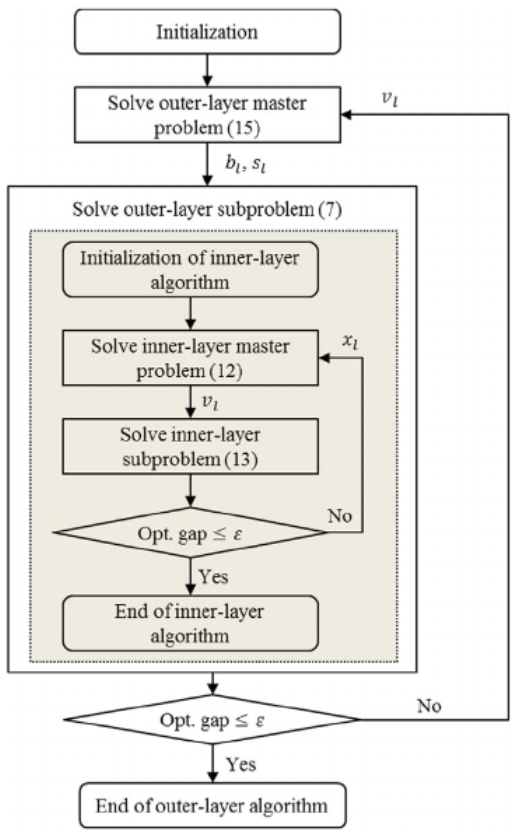
Engineering antifragility of critical infrastructure
A system is antifragile if its performance improves as the result of exposure to stressors, shocks, or disruptions. This behavior is typical of complex systems and is not usually exhibited by engineered technical systems. In fact, technical systems can display antifragility when new investments are allocated, e.g., after disasters. We have developed an optimization model for the post disaster restoration planning of infrastructure networks, taking into account the possibility of combining the construction of new components and the repair of failed ones. The strategic goal is to determine the optimal target system structure so that the performance of the target system is maximized under the constraints of investment cost and network connectivity. The proposed approach is tested on a realistic infrastructure network: the 380-kV power transmission grid of northern Italy. The results show that the restored network can achieve an improved functionality as compared to the original network if new components are constructed and some failed components are not repaired, even when the former is much more expensive than the latter.
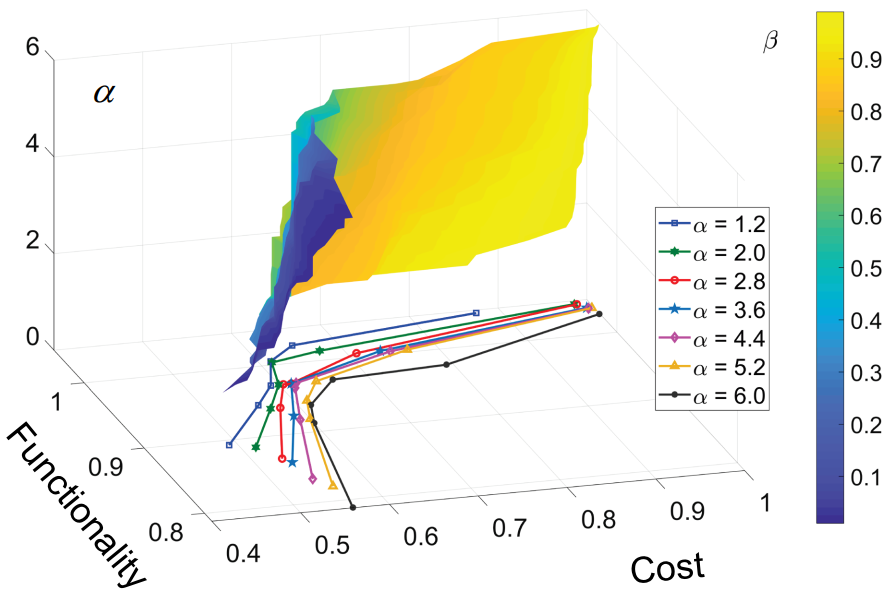